As generative AI (GenAI) continues to grow, educators are finding new ways to navigate or integrate it into their teaching while upholding academic integrity. Educators are modifying assignments, adapting assessments to focus on critical thinking and uniquely human skills, and discovering ways to enhance learning through GenAI.
Early AI models made a lot of mistakes, making GenAI use obvious. It was easier to ask students to acknowledge GenAI use and take responsibility for errors. Newer GenAI models produce fewer mistakes, so this approach is becoming more complicated. Allowing GenAI in course activities without clear intent and structure risks unintentional and deliberate academic misconduct and missed learning opportunities. This blog provides an overview of strategies for addressing GenAI in assessments and introduces a model for redesigning rubrics to clarify expectations.
Strategies for Addressing GenAI in Assessment
Require students to complete assignments without access to GenAI.
In-class activities are a strategy for situations where GenAI use does not benefit learning. This might include discussions, quizzes, midterms, and invigilated exams. However, handwritten work and restricting technology can pose accessibility challenges and hinder universal learning (UDL).
Motivate students to complete assignments without misusing GenAI.
This approach is about intentionally getting to know learners as individuals, regardless of the course modality. Plan formative assessment or diagnostic activities early in the course to find out what learners already know about the content, and to gain familiarity with their writing and voice. This could be done with a small piece of writing, a Teams call, or a one-on-one interview, to establish a baseline that can be referenced later should you suspect GenAI misuse. Generally, there are underlying issues at the root of academic integrity such as time management, inexperience, or personal issues. Instructors can prevent perceived learning shortcuts by understanding learner needs, by helping students navigate the course, and by connecting learners to support services such as the writing center, tutor support, student support and intervention and other services.
Collaborate with GenAI to build literacy.
Educators can design assignments that emphasize the learning process rather than just the final product. For example, in writing tasks, GenAI can be strategically integrated as a tool for content generation, allowing students to focus on critically evaluating and refining information. This positions GenAI as a partner in learning while helping students develop essential skills for assessing accuracy, reliability, and potential biases in AI-generated content. What specific skills do you want students to develop to make informed decisions about the content they use?
GenAI can also support diverse ways for students to demonstrate their learning. For instance, students who struggle with writing might use AI to generate an outline or brainstorm ideas. Those who excel in visual communication could represent their learning as an AI-assisted infographic or video script, making the learning process more inclusive and personalized.
Design assignments to disincentivize GenAI use.
Rethinking traditional assessments can reduce reliance on GenAI. Instead of standard exams or essays, consider alternative assessments. Could an exam be replaced with a project? Could students record their responses as audio or video?
To make assignments more effective in measuring student learning, test your writing assignments by entering them into GenAI tools like Microsoft Copilot, ChatGPT, or Google Gemini. Evaluate where AI-generated responses meet expectations and where they fall short. Then, modify the assignment to emphasize key learning outcomes, focusing on skills that require critical thinking and originality. Conversely, minimize assessment activities that GenAI handles well, such as summarizing, generating outlines, paraphrasing or correcting grammar.
Use a combination approach: incentivize the learning outcomes.
This approach integrates building relationships with learners as described above and helping learners see the value in the assignment. Involving students in assessment design, such as co-creating rubrics or choosing project formats, also builds ownership and motivation. Incorporating empathy and community-based learning allows students to see how their work impacts others, making learning more meaningful. Educators can design assessments that highlight the value of the learning process and incentivize learning outcomes through the grading scheme or rubrics.
Redesigning Rubrics
Rubrics can be used as a means of communicating expectations for students on how to, or how not to use GenAI in assignments. Rubrics can also help prevent confusion and disputes about the grading of assignments by providing a standard set of expectations for all learners within the course. Rubrics can emphasize qualities that are difficult for AI tools to replicate. For instance, revise rubrics to prioritize authenticity and references. Effective rubric criteria might include the following:
- Critical Thinking: The work reflects originality and significant critical thought.
- Authenticity: The response communicates the student’s ideas and perspective in an unscripted, personal way.
- Reference: The response engages directly with course materials, citing relevant texts, lectures, or resources, and demonstrates a clear understanding of disciplinary concepts.
- Organization: The response is accurate, well-structured, addresses all parts of the assignment, and follows the guidelines.
A Model for Rubric Re-Design
Amanda Brijmohan from the University of Toronto, suggested a four-part model for rubric re-design as a guide to integrate generative AI into teaching and assessment practices.
- Instructor Familiarity with Generative AI: Encourages educators to assess their understanding and experience with generative AI tools, as this will influence how they design assignments and rubrics.
- Learning Outcomes: Focuses on identifying the knowledge and skills that the rubric criteria aim to assess. It also considers whether additional criteria are needed if generative AI is incorporated into assignments.
- Rubric Development with Generative AI Use: Guides the redesign of rubrics to account for the integration of generative AI, ensuring that expectations for AI use are communicated. The Artificial Intelligence Assessment Scale (AIAS) is a tool to support communication.
- Rethinking Assignment Components: Encourages educators to reflect on what they want students to learn from assignments and how generative AI should or should not be used in their classrooms. This includes considering how assignment submissions might change and what additional learning artifacts (e.g., AI chat logs, reflections) students may need to provide.
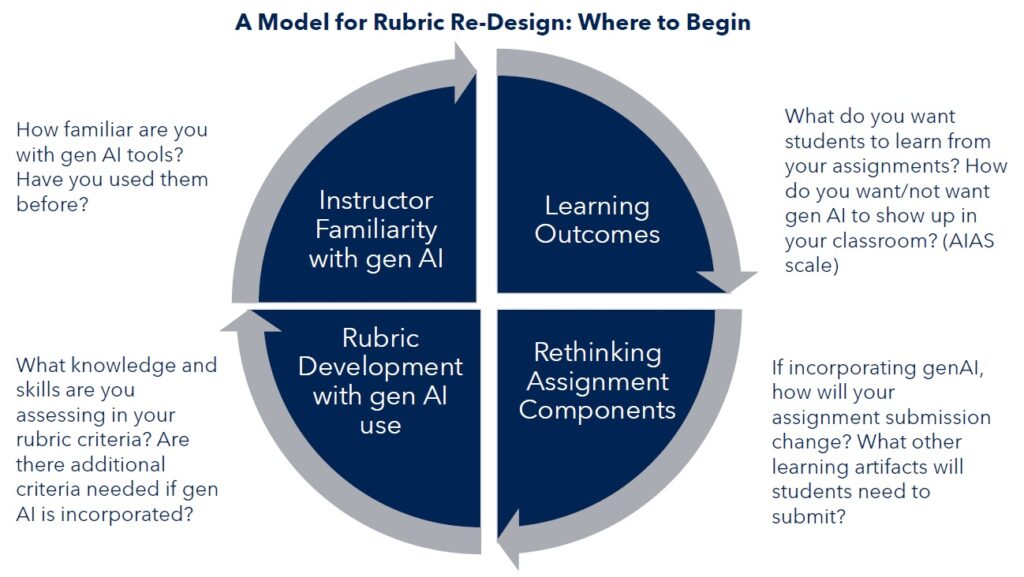
Example Rubrics
To see example rubrics, review the Rethinking Rubrics in the Age of Generative AI document (pages 16-21) and watch the AI-Proof Your Course in 4 Steps video (the rubric examples are around the11.5-minute mark).
As teaching and learning continues to evolve alongside GenAI, taking time to reflect on your approach can help create meaningful learning experiences. If you are rethinking assessments, exploring new approaches, or wanting to learn more about GenAI tools, the Centre for Innovation and Excellence in Learning (CIEL) invites you to connect with us. We are here to support you.
Attribution
Brijmohan, A. (2025). Rethinking Rubrics in the Age of Generative AI. https://www.utm.utoronto.ca/rgasc/media/3442/download?inline
Elkhoury, E. (2021). A guide to alternative assessments. https://www.yorku.ca/teachingcommons/wp-content/uploads/sites/38/2021/07/Guide_alternative_assessments-UPDATED-July-2021.pdf
Perkins, M., Furze, L., Roe, J., & MacVaugh, J. (2024). The Artificial Intelligence Assessment Scale (AIAS): A Framework for ethical integration of Generative AI in educational assessment. Journal of University Teaching and Learning Practice, 21(06). https://doi.org/10.53761/q3azde36
Pixelated Pedagogy. (2025). AI-Proof Your Course in 4 Steps. https://youtu.be/MaIHfnd8hpI
Washington University in St. Louis. (2024, July 31). AI resistant assignments. Center for Teaching and Learning. https://ctl.wustl.edu/resources/ai-resistant-assignments/